World of methodologies | First peek
This series of featuring blog posts aims to open the world of data analysis methodologies. The goal is to bring the insight of data scientists onto Real-World Evidence (RWE) studies and Real-World Data (RWD). Like in the main blog series, the goal is to keep a high-level view of the topics at hand, without any need for prior knowledge on the issue – even when the context is complicated. Because the writers possess technology-oriented backgrounds with expertise in coding and statistics, we do our best to avoid over-complication. However, speaking in layman’s terms is not necessarily our strongest suits (especially according to stereotypes); proceed at your own risk. The contents for these featured posts will be mainly written by Medaffcon’s data scientists. The author of this first post is Iiro Toppila, the Data Analysis Lead of Medaffcon.
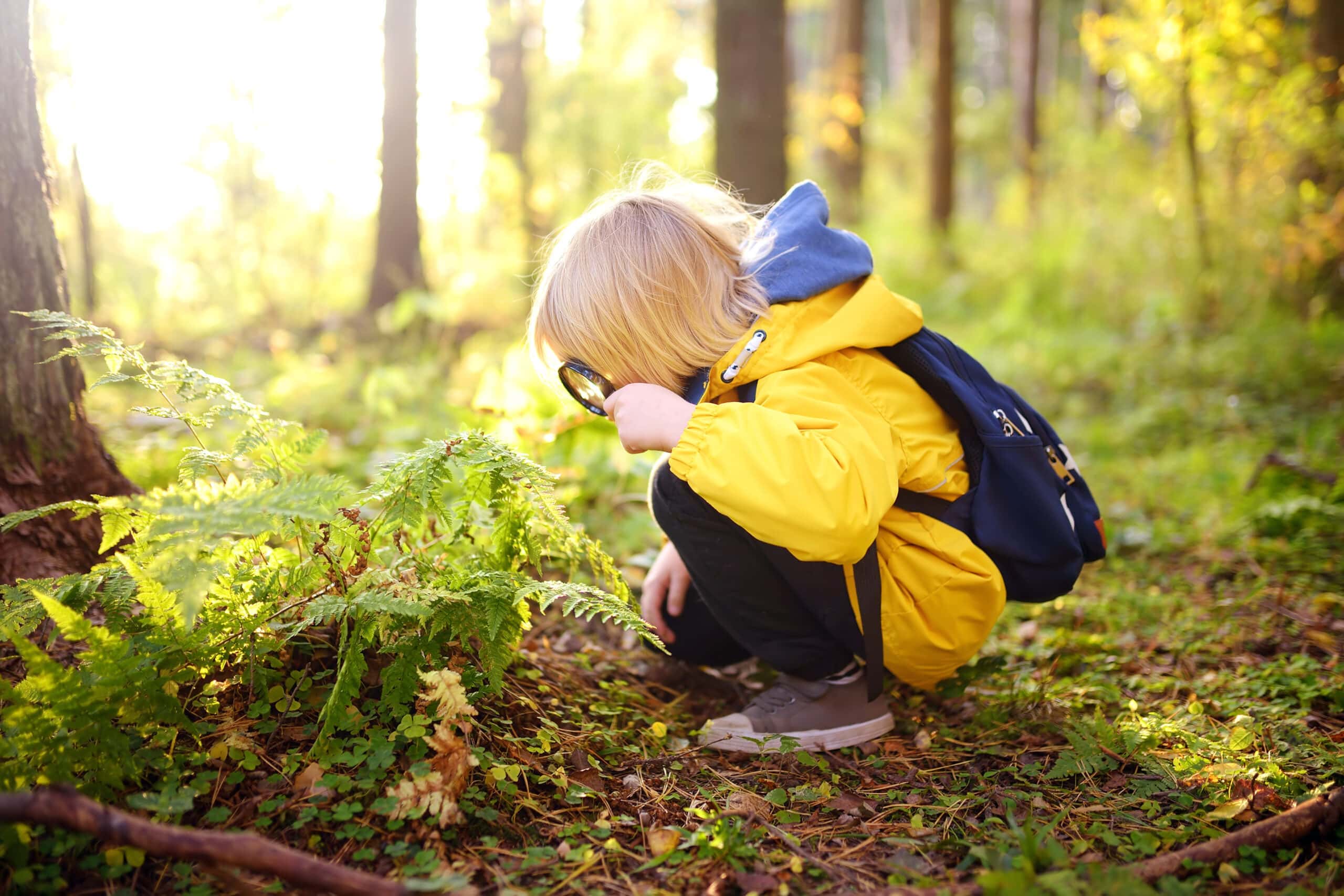
To make a great RWE-study, you need a well-formulated study question, Real World Data of the highest quality, as well as an excellent executive study team. In short, the process aims to squeeze information from the data using carefully selected methods. The main goal of these featured blog posts is to familiarize the reader with the spectrum of various methodologies one might encounter in one’s journey with the RWE studies.
The methods used in RWE-studies can mainly be classified as “traditional statistics”. However, for all settings and questions, there are no suitable methods in this toolbox. One of these things not fitting this box, which has gotten a lot of attention lately, is machine learning and artificial intelligence (AI). Even though these might sound scary and complex as words, they are mathematics where traditional statistics are. And in some settings, machine learning and AI are nice and well-suited tools also in RWE-study settings.
On terminology
To be strict, machine learning and AI are two distinct things. Machine learning is a subcategory under AI. But let’s not go into the details. Especially in layman’s terms and in marketing, these two are interchangeable. Generally, defining AI is closer to a philosophy where machine learning is just applied mathematics.
Machine learning can be used when discussing “systems” that can learn and advance based on their experiences without being directly programmed on the task. AI is intelligent acts and decisions demonstrated by machines – even though they would be directly preprogrammed (such as older chess programs).
Of these two, we focus more on the actual machine learning methods. However, the term machine learning could be replaced with “AI” practically throughout the text if you prefer, especially as we are discussing things on a higher level and using layman’s terms. You get the point.
From history to today
The theory behind machine learning is old. Very old. The math behind it originates from the ancient times before computers. However, when moving to the 2010s, machine learning has gone through many breakthroughs and advancements, partially driven by the ever-increasing computational capacity of computers.
The most significant advances have been achieved outside the health cluster, in fields where there is more data, and it is more accessible. The hype around AI is gradually starting to calm down. Today, just having a sexy AI component included in a product is not a value itself.
Still, machine learning is currently trending in the health cluster. Machine learning as a field has advanced to a point where the methods and their limitations are understood, at least to some extent. Especially, now we are starting to understand what types of questions might be solvable using machine learning and what is not necessarily worth the effort.
Machine learning and RWE
But does this have anything to do with RWE-studies? Actually, yes, quite a lot.
First, most machine learning methods have one common feature: they need lots of data and examples (at least compared to traditional statistical modelling). The more complex the question, the more data is required.
Generating health data is also relatively slow and expensive. For example, if one would like to develop AI to recognize osteoarthritis, collecting thousands of cat scans from knees would be a slow and costly process, especially compared to facial recognition AI and the cost or time required to collect thousands of selfies for a corresponding dataset. Sounds like a suboptimal setting.
However, RWD generated in routine care, and the legislation to utilize it for secondary uses provides a solution for the dilemma. You do not need to generate the mountains of data; it is already there (and more is generated every day).
Second, Real-World Data is the optimal base for machine learning when you want to solve real-world problems. The machine can only learn things that exist in the data presented for it. Therefore, if the data would come, for example, from clinical trials (where they have strictly selected subpopulation of the Real-World patients), the predictions by the machine would not necessarily be applicable in a real-world setting. The RWD does not select individuals, and it presents things as is – without any additional sugar-coating – exactly as the attending clinician originally recorded them.
In conclusion
Generally, machine learning and artificial intelligence have lots of potential uses in healthcare – in principle. In RWE-studies, the potential of these sophisticated advanced analytics is overlooked more than often.
In the following featured blog posts, we will dive deeper into some methodology families, including some from “traditional statistics”, unique features of different types of data, and discuss practical examples of how these all can be utilized when working with RWE data. Naturally, using layman’s terms.
While waiting for the future “world of methodologies” posts, some of the impatient ones can, for example, have a sneak peek at the basics of machine learning with an open-access course “Elements of AI” provided by Reaktor.